5 Lessons on Digital Experiments From the Experts
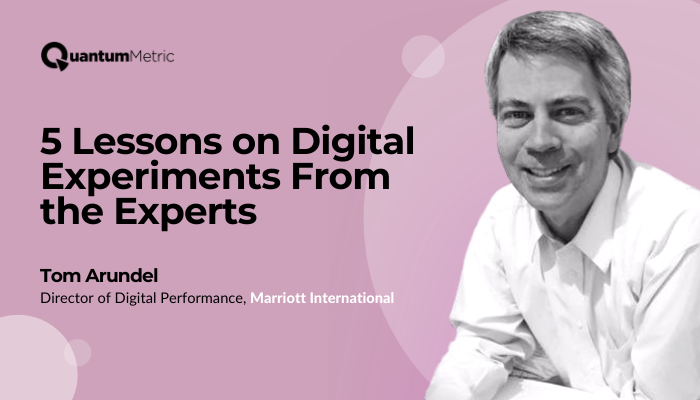
This guest post is part of our Continuous Product Design (CPD) Evangelist series.
Tom is Director of Digital Product Performance at Marriott International. In this post, Tom Arundel shares lessons on digital experiments from his own experience and interviews with two experimentation experts, Lee Carson and Martin Aliaga.
We learn more from our failures than our successes. That’s because we’re less likely to repeat mistakes if we’ve paid the price. For years, digital experiments have been a great way for companies to learn from lots of mini failures without risking the bottom line. They can fail forward.
In my career, I’ve had the opportunity to work as an analyst on a few A/B tests as well as work alongside some of the most talented leaders in digital experiments and optimization. I can share my experience as well as the wisdom of two gurus who have seen the trenches of digital experiments for top digital organizations.
I spoke with Lee Carson, VP of Platforms at Waybetter Marketing, and Martin Aliaga, Director of User Research and Digital Experiments at Marriott International. They shared best practices and insights from their years of running successful digital experimentation programs.
The difference between experimentation and personalization
First things first, let’s clarify what we mean by digital experiments. As the technology that powers experimentation accelerated several years ago, marketers began to blur the lines between personalization and experimentation, but there is a difference.
Personalization, which is often driven by machine learning and predictive algorithms is a kind of “hyper-optimization” that allows your entire website to be customized to visitors based on data about their customer profiles, loyalty indicators, products viewed, stage in the customer journey or traffic source. Innovators like Quantum Metric use machine intelligence to personalize an experience based on real-time behaviors and struggle indicators.
Digital experimentation, also known as A/B testing, multi-variate testing or optimization, is more limited in scope, serving up two or more alternate experiences on a page (i.e. variations of an image, button or link) to a majority of users, based on a predefined hypothesis. The goal is to pull data about user behavior to measure if version A or B performs better. Typically, an experiment is live for all visitors to a page or may take into account traffic sources to the page.
In short, website personalization depends on understanding your users at a deeper level and is carried out with the user profile in mind. Digital experiments are typically highly focused on improving one area of the site and rely on a hypothesis. Neither of them is a substitute for the other, and both reserve an important place in your digital transformation.
In this post, we identify some of the key learnings around building and running digital experiments, from test design and hypothesis to test results and analysis.
1. Leverage your data layer
Since data is at the heart of any digital experimentation program, make sure your website has a foundation for capturing and mapping to user data. Two ways to achieve this are through the data layer and CSS selectors.
The data layer is the behind-the-scenes library (or dictionary) that your digital experiments can tap into for real time and consistent data about transactions, product attributes and customers. To build a universal data layer, you’ll likely need to work with IT teams. As you run new experiments to test average order value or conversion rates, the data layer provides a single object to easily map to. This ensures that a product name (for example) conveyed in your web analytics tool is the same name conveyed in your digital experiments tool.
In a perfect world, the data layer is nice, but it isn’t always available for what you need to test. There are workarounds to avoid the data layer being a bottleneck to A/B testing. As an alternative, use CSS selectors to target elements in your application. A CSS selector is a string used to identify and locate one or more elements on the page. It often uses a consistent naming structure, which makes it easy for JavaScript-based testing tools to identify and access.
2. Develop a data-driven hypothesis
It’s easy to fall into the trap of creating “hunch-driven” hypotheses, based on qualitative data or worse yet, internal stakeholder or executive input based on observing one bad experience. “It’s not uncommon for a product manager to have developed a new feature or a marketer just wrapped up the finishing touches on a new campaign,” says Carson. “But some VP doesn’t like an aspect of it, and suddenly an A/B test is born.”
To drive more meaningful experiments, isolate the actual problem and develop the hypothesis based on quantitative data from real customers using analytics, not input from VPs and stakeholders.
Take the guesswork out of isolating the problem by understanding where users underperform and prioritizing the issues that cause real friction. “There are times when product teams are very eager about improving an experience without clearly identifying the problem,” says Aliaga. “If you can’t articulate the problem and validate that it’s an actual problem, then it’s difficult to create a sound test strategy. You should use data to confirm the problem exists and size the potential impact of making an improvement. Sizing the potential impact helps when it comes to prioritization.”
3. Design multiple iterations around possible edge cases
An A/B test isn’t always just about testing versions A and B. You may need to design multiple experiences and iterate on tests frequently to find a true winner.
First, as you prepare to set up and design the experiment, think through the entire user journey. “Many of us assume a user is navigating our site in a very linear manner,” says Aliaga. “We need to think through the entire experience to address any edge cases for users that are entering a test from different paths. Doing so may elevate unexpected issues in the experience.”
Second, you may need to design a multivariate test with several rounds of testing in order to move the needle. “Based on low win-rate (the percentage of experiments that achieve a positive statistically significant result), you can expect it to take 5 to 10 experiments to find a true winner,” says Carson. “Try to run experiments with 3-4 experiences and then plan for it to take 2-3 rounds of testing to find revenue.”
Moving the needle isn’t easy. But don’t give up too early if you’re not seeing positive results. “Most experiments are designed around the assumption that it’s going to drive revenue,” says Carson. “There is a strong body of evidence that well-designed websites have a 10-15% win rate against such north star metrics. You are more likely to see significant results in step metrics like clicks, but those micro-conversions do not always lead to revenue. The more you experiment the more you realize that it’s pretty easy to convince someone to click a button, but it’s a lot harder to change someone’s underlying motivation.”
4. Validate pre-launch and communicate proactively
In the excitement of building and launching a test, there’s a tendency to rush to production before everything has been validated against the rest of your site’s functionality. In large, complex digital organizations especially, experiments are just one part of a living, breathing digital ecosystem that’s continuously updating. Be aware of any competing product or platform roadmaps with changes taking place at the same time as your A/B tests.
“Unanticipated platform or site changes can break the test experience,” says Martin Aliaga. “In order to avoid this, you need to be very proactive with the IT teams that are making platform updates. Do your best to understand when these changes are taking place. Many times, these experiences are live in a dev environment. Be sure to QA your test in the dev environment to ensure compatibility.”
It’s also important to communicate changes as they go live. “Get feedback from internal stakeholders who may be unaware of the test,” says Aliaga. “Communicate broadly when a test experience goes live and provide details about what is changing and the length of the test. Keeping all stakeholders informed is a good practice.”
5. Avoid snap judgements
In the first few days of a test, you might see either a strong negative or positive result and have a tendency to call it a winner or loser. Make sure you have a valid sample size and thorough test duration to achieve statistically significant results. Use other analytics tools to validate user behavior during the test. Even if one segment wins, it doesn’t mean customers aren’t still frustrated. And it’s important to remember that the smaller the difference in conversion rate between two test variations (A and B), the greater the sample size that’s needed for the test. This means running the test longer, especially on lower traffic pages, to ensure a valid sample size is achieved.
“When you see exceeding positive or negative results from an experiment the first couple days the temptation is to call the test early and roll it out to full traffic or kill it before it can do any more harm,” says Carson “Do check for technical errors, but if you don’t see any, let it run. The results will most likely come back to earth. If you want to call experiments early, you’ll need to change your statistical methods to accommodate and that usually means hiring a statistician.”
The experts highlight three ways to avoid snap judgment when analyzing results:
First, run the recommended duration. Acting on the data too prematurely is a common mistake according to Aliaga. “Always allow the test to run through its full test-duration. You don’t want to introduce any bias by calling a winner too soon.”
Second, look for unintentional red herrings. As the test comes to an end, it’s important not to take results at face value. “If the results are too good to be true, they probably are,” says Carson. “At the very least, they may not mean what you think they mean.”
Three examples of “too good to be true” test results include:
- You are testing a three vs. four-page process. The four-page process is seeing massively positive results. After the test, you realize the conversion tracking pixel is missing on default experience, making the alternate experience appear to have a more positive result. As an added precaution, compare data from your experiment with your day-to-day analytics. By comparing page view counts, you should be able to confirm results may in fact be too good to be true.
- You are testing two hero images and the creative you like wins. After the test, you realize the winning image was optimized, but the alternate came in at 2 MB. To prevent jumping to conclusions, compare your experiment data to page speed and performance analytics.
- A subject line increased lifts in opens and clicks. After the test, you realized that the extra clicks were coming from bots analyzing the spam word you put in the subject. Your email was getting caught up in spam filters and the number of humans clicking when down. To avoid this, break down your top-line results to understand which segments were affected and how. It should be pretty easy to see the impact of spam filters once you break out bot-influenced traffic.
Lastly, be rigorous and apply discipline when calling winners. There can be an overwhelming number of metrics to look at when you run a digital experiment, but only a few that make a difference. “Assuming the test was sized properly, then focus on the primary success metrics,” Aliaga states. “It’s important to look at step-conversion and secondary metrics. However, if you drill down to too many metrics you can get conflicting signals. Remember what metric was used to size the test duration. Keep in mind that the test may not have been sized to measure the lift to a subset of audience segments and metrics.”
Summary:
Digital experiments allow you to gain reliable insight and continually fine tune every aspect of the customer journey. They take the guesswork out of design and allow you to consistently deploy compelling experiences. However, it’s important to build a program around continuous improvement and optimization, with the understanding there will be winners and losers. “Many large organizations run up to 100 experiments per year and that translates to 10-20 winning experiments,” says Carson. “Think of your experiments as part of an ecosystem of learning as opposed to one-and-dones.”
Be sure to ask the tough questions and remember that experimentation is about failing fast. “Don’t assume the test experience has to be 100% polished,” says Aliaga. “In the spirit of failing/learning fast, it’s better to get a test out in market to collect real data. Testing is an iterative process. You can leverage the findings of an initial test to inform a more vetted/polished test experience.”