Why data sampling in experience analytics is a limitation.
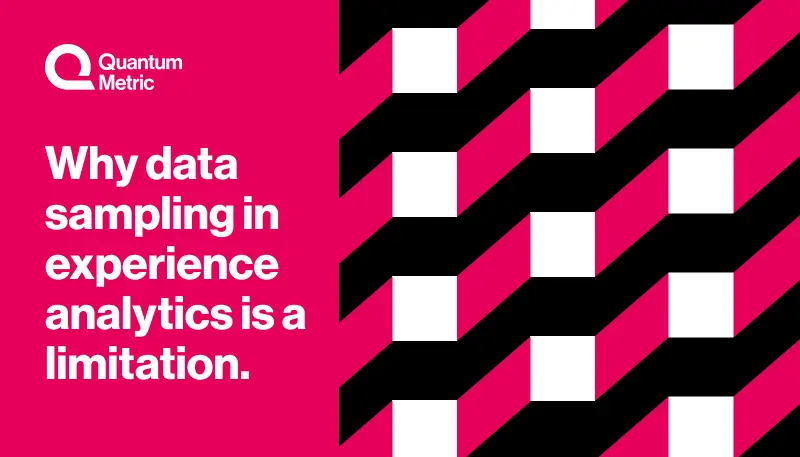
What is sampling and why does it exist?
The year is 2006. Justin Timberlake’s “SexyBack” tops the music charts.
Organizations are storing their data on-premises and cloud providers are emerging, and it is all still a bit expensive. This environment shaped the analytics providers of the day.
Traditional web analytics providers are typically priced by the volume of data that they capture. Session replay vendors limited capture to reduce performance overhead and storage costs. In short, they sampled. Whether a certain part of the digital experience (e.g. just the checkout pages) or a certain percentage of your audience (e.g. 15% of sessions), sampling was frequently utilized.
This meant there was a cost to visibility, because there was a significant cost for storage and the margins didn’t make sense for the vendor or the buyers to do anything differently.
The data explosion and the emergence of the cloud.
The good news: Things have changed, rapidly. Storage costs have gone down and cloud emerged as the preferred option. Also, data collection methods and experience analytics capabilities have matured significantly.
On top of that, organizations have digitized—some seemingly overnight. Organizations have complex digital experiences from websites, to mobile apps and kiosks. To be competitive they can’t just “work,” they have to be predictable, delightful, and easy. So this means that leading organizations have invested more in analytics to understand their customer’s experience.
A new category of technology has emerged, known as experience analytics. This category of technology uses a combination of session replay, heatmaps, and machine learning driven analytics to help organizations identify optimization opportunities across their digital experiences.
So with this evolution in customer expectations and the rise of new technology, sampling is a relic of the past right? Not exactly.
Why are we still sampling?
Session replay has been through multiple revolutions. Many of these have improved performance, lowered overhead, and improved security. But these revolutions have not been standardized across the industries.
Many providers have acquired legacy technology solutions that have heavy overhead. Customers, and especially large customers, won’t be happy with the performance hit that comes with capturing at 100%. So how have these vendors adapted to less than ideal technical debt? Sampling.
Why you should demand complete data visibility.
Let’s talk through some of the downsides of taking the sampling approach.
1. Incomplete analytics.
The first and most obvious is poorer analytics resulting from a sampled dataset. Sampled data can be useful for directional and high level segmentation data. For example, you can likely have a fair bit of accuracy when it comes to understanding if an audience is mobile web or desktop web. Even if only capturing 10%, you can extrapolate out of that with a fair degree of accuracy.
The sampling approach falls short when advanced segmentation and web analytics come into play. Modern experience analytics providers will leverage machine learning and alert on very specific errors impacting particular segments. When you sample, you automatically reduce the number of sessions in narrowed segments, and when n is small, statistical reliability and anomaly detection capabilities are reduced.
Example: Let’s say you have about 1 million sessions a day. You are sampling at 10%, so you are capturing about 100k sessions a day. Then you start layering on segments like regionality, device, and browser which further reduces the sample. An error you were looking for is occurring in 5% of overall sessions. It quickly becomes clear that this is not enough data to declare if this is a “real” issue or not.
At large organizations this is a problem because they are frequently aware of the issues that are dramatically impacting top level metrics like conversion rate, revenue, or task completion. Experience analytics typically helps them get to the root cause and if the issue is widespread enough, a sampled dataset can even suffice. It’s the discrete issues, where maybe a specific segment of your audience (like described above) is running into friction. With sampling, it’s infinitely harder to pull these needles from a haystack.
Furthermore, for platforms that do forward looking opportunity analysis to understand business impact, sampling poses a risk. For example, let’s say a segment of your audience experiences an error (let’s say a slow loading page which is causing them to force reload the page) and are converting at a lower rate. To help you quantify the impact of this issue, modern platforms will compare this segment against the norm, look at average value per customer, and help you calculate the “cost of doing nothing” when it comes to fixing this particular friction point. This works great when you capture 100% of traffic. When you sample it’s much easier for outliers to emerge, making it harder to trust (and act on) the data.
2. Session replay loses its utility with sampling.
One of the many reasons that session replay is so valuable is simply speed. The speed to “aha!” is unmatched. You can comb through VoC responses, stare at dashboards, and dig through log files for hours. Within seconds, a session replay can tell you what happened and why.
So if you capture only a portion of replays, everything gets harder.
Example: Imagine that your CEO calls you. She tells you that she just had a horrible experience on the newly redesigned checkout page. Fix it. What’s next? Ask her to walk you through every step she took? Or pop open the browser console and read off the JS errors she is seeing? Of course not. You say you’re on it and head over to session replay to see what happened. But what if her session wasn’t captured? Nobody wants to be there.
Session replay is valuable because it guarantees a level of empathy and understanding with every individual customer experience. Without having every replay at your fingertips, teams will scatter trying to piece together their idea of what happened. This doesn’t happen when you capture 100% of session replays.
This isn’t just for executive escalations too. Anytime a customer complaint comes in, your customer support team needs to be able to pinpoint the exact issue, every time.
3. Contact center use cases vanish.
As we’ve stated, session replay technology is rapidly evolving. So much so that many top replay providers are capturing session replays in real-time. This real time understanding of the customer experience opens up a host of use cases. Customer support agents can now empathize with their customers by pulling up the session replay as it’s happening. Oftentimes, they can do this directly in their day to day tools like Salesforce Service Cloud.
And with contact centers, there can’t be unpredictability. An agent has to be able to rely on their tools, so a sampled dataset isn’t an option in contact centers.
Contact centers are all about efficiency. Incremental improvements (or hindrances) can drive huge business outcomes. For example, if an agent can reliably look up a customer session for every call, that can help them do their job. It can give them more context, help them empathize, and at the end of the day create a better outcome for the customer (and the agent). But if they can pull up Customer A’s session but not Customer B’s, it breaks the process. Agents won’t be able to find the customer and this lack of predictability would prevent something like session replay from being used in the call center.
100% capture is just the start.
Being able to capture 100% of sessions with low overhead is just the start. It’s one of the many critical areas you should use to evaluate session replay and experience analytics providers.
You can find this criteria in our Experience analytics buyers guide, a straightforward guide to evaluating the rapidly expanding experience analytics market.